Machine Learning Applications in Business and Beyond
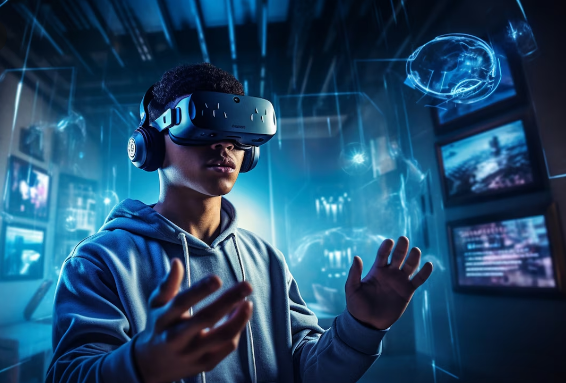
Machine learning (ML) has emerged as a transformative technology with diverse applications across various sectors, revolutionizing how businesses operate, make decisions, and innovate. At its core, machine learning involves the development of algorithms that enable computers to learn from and make predictions or decisions based on data, without explicit programming. This capability has fueled advancements in areas such as customer insights, predictive analytics, automation, and personalized recommendations, reshaping industries and driving unprecedented growth and efficiency gains.
Machine learning’s ability to analyze large datasets and extract meaningful patterns and insights has made it a cornerstone of modern business operations. In marketing and sales, for example, companies leverage ML algorithms to analyze customer behavior, preferences, and purchasing patterns. By identifying correlations and predicting future trends, businesses can personalize marketing campaigns, optimize pricing strategies, and improve customer retention through targeted recommendations and promotions.
Moreover, machine learning plays a crucial role in enhancing operational efficiency and decision-making across supply chain management, logistics, and inventory optimization. ML-powered algorithms analyze historical data, demand forecasts, and external factors to optimize inventory levels, streamline distribution routes, and minimize transportation costs. These applications not only improve supply chain resilience but also enhance responsiveness to market fluctuations and customer demand.
Beyond traditional business functions, machine learning is driving innovation in healthcare, transforming diagnostics, personalized medicine, and patient care. ML algorithms analyze medical imaging data, such as X-rays and MRI scans, to detect anomalies and assist healthcare professionals in early diagnosis and treatment planning. Additionally, predictive analytics models based on patient data enable healthcare providers to anticipate and mitigate risks, reduce hospital readmissions, and improve overall healthcare outcomes.
In financial services, machine learning algorithms analyze vast amounts of transactional data to detect fraudulent activities and protect against cyber threats. These algorithms continuously learn and adapt to evolving patterns of fraudulent behavior, enabling financial institutions to enhance security measures and safeguard customer assets and sensitive information. Furthermore, ML-based algorithms power automated trading systems that execute trades based on real-time market data and predictive analytics, optimizing investment strategies and portfolio management.
The transformative impact of machine learning extends beyond traditional sectors to areas such as agriculture, where ML algorithms analyze soil conditions, weather patterns, and crop health data to optimize farming practices and maximize crop yields. By leveraging predictive analytics and precision agriculture techniques, farmers can make data-driven decisions on irrigation, fertilizer application, and pest control, thereby improving productivity and sustainability in food production.
Looking ahead, the future of machine learning holds immense promise for innovation and societal impact across various domains. Advancements in natural language processing (NLP) and conversational AI are enabling more intuitive human-computer interactions, revolutionizing customer service, virtual assistants, and language translation services. Furthermore, the integration of machine learning with emerging technologies such as edge computing and Internet of Things (IoT) is enabling real-time data processing and decision-making at the network edge, enhancing efficiency and responsiveness in autonomous systems and smart cities.
In conclusion, machine learning applications continue to redefine business operations, drive innovation, and empower organizations to harness the power of data for strategic decision-making and competitive advantage. As machine learning technologies evolve and become more accessible, addressing challenges related to data privacy, algorithm bias, and ethical considerations will be crucial to maximizing the benefits and ensuring responsible deployment of AI-driven solutions across industries. Machine learning’s transformative potential to solve complex problems, optimize processes, and improve human lives underscores its status as a cornerstone of the digital economy and a catalyst for future innovation and growth.